The key technology behind Neural Alpha's Disclosure Assistant

The key technology behind Neural Alpha's Disclosure Assistant
Large language models (LLMs) are getting better at basing their responses on hard facts. In the past they have been prone to hallucinations that have made their responses unreliable at best and catastrophically misleading at worst. Using a LLM to provide support for business decisions would until recently have been a high-risk strategy.
Recent innovations have made LLMs less likely to hallucinate given enough context alongside a prompt. One of these innovations is called Retrieval-Augmented Generation (RAG), a way of adding factual context to a prompt at runtime that makes the response more grounded in reality.
Our Responsible Capital Disclosure Assistant product uses this technology to enable lenders and investors to analyse portfolio companies and their investment universe when performing ESG Assessments at scale for comprehensive frameworks such as TNFD. Structured datasets constructed using our toolkit can be used to rank and score companies and to develop bespoke internal ESG ratings if desired. Corporates are also using the toolkit for baselining their own corporate sustainability initiatives and disclosure approaches against competitors by systematically assessing industry peers’ performance for different sustainability facets.
How Neural Alpha is using Retrieval-Augmented Generation
We're used to LLMs being able to produce a plausible response to specific questions but we're not always sure where the LLM got its information from or whether it simply made something up that sounded good. Now, though, we can encourage the LLM to admit when it doesn't know something and also back up its assertions with useful citations.
The way we're doing this is by building a Knowledge Base of known facts about a company's products, activities, policies, and other content, each accompanied by a text snippet - a quote from the company's own disclosures. Because this is stored in a vector database we can easily find the snippet that most closely answers the question put to the LLM. Disclosure volumes are already prohibitive for investors and will continue to grow exponentially illustrating the value of extracting the most relevant facts from an entire universe or portfolio of companies.
Using these chunks of highly relevant and contextualised information we can ask the LLM to answer any question and be sure that the response is both grounded in the company's own corpus of documents with the provenance of assertions retained by including references alongside answers.
This way we get the benefits of the language skills native to a LLM plus the factual information disclosed by companies combined in an authoritative response to any assessment question.
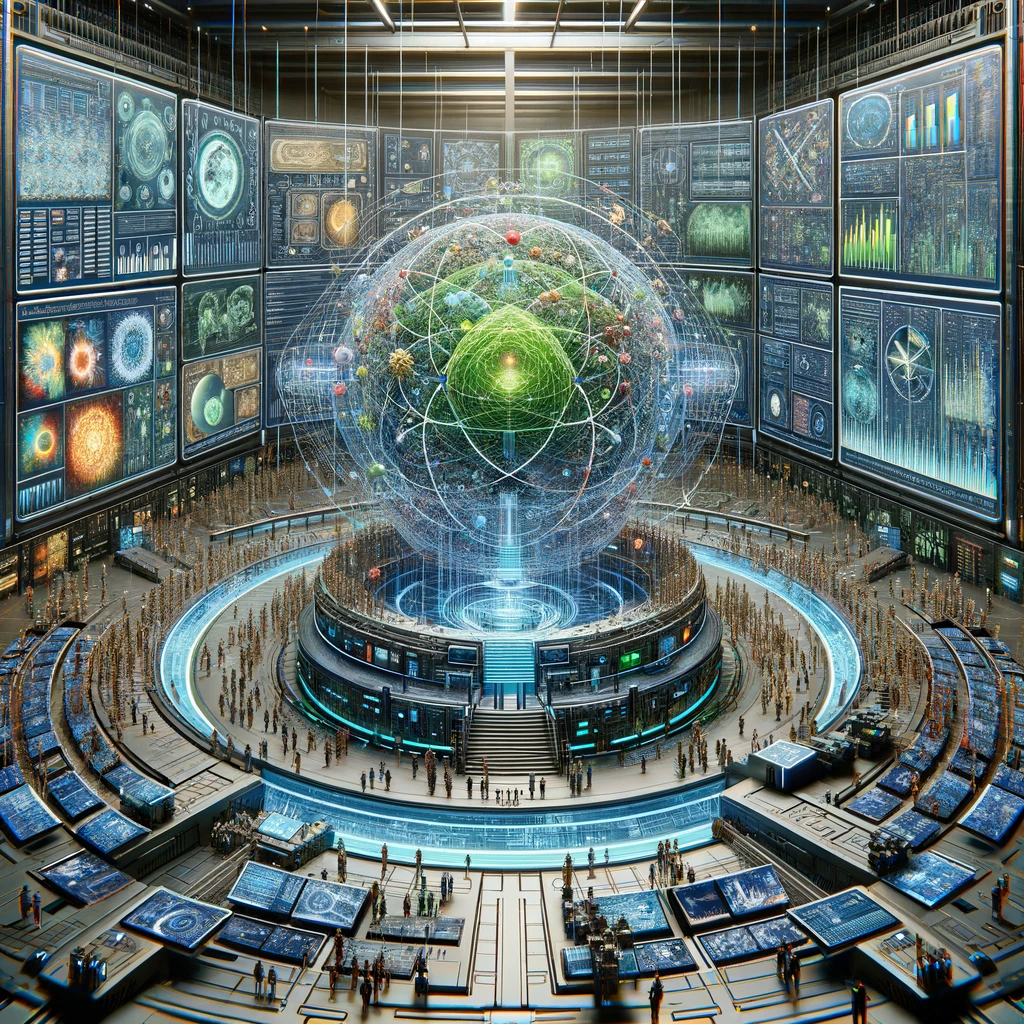
Our ESG Knowledge Base helps to guide Large Language Models (LLMs) to perform targeted assessments and return contextualised answers
Vector databases, embedding models, cosine similarity and semantic proximity
We use a vector database because this is the natural data store for an embedding model. It's the embedding model that does the useful work of transforming unstructured data into a numerical representation for us to store in the database.
We do this transformation first for our source documents, creating a landscape of components for our response, based on the disclosure documents we have found. We then transform the prompt issued by a user in the same way.
We end up with the prompt finding a home in the landscape of snippets and we need to find the most useful snippets to associate with the prompt. This essentially means finding its nearest neighbours in the landscape and for that we use cosine similarity, a mathematical tool for measuring both distance and direction in a multidimensional vector space.
With these techniques we can find the appropriate fact-based text snippets for any question asked of our data.
Use cases and early adopter experience
We’ve tested our disclosure assistant on a wide range of Use Cases to date and are immensely grateful to all early adopters who have provided valuable feedback to help us improve the product. So far interesting use cases have covered a range of areas within finance including investment research, risk management, ESG assessments, portfolio screening, universe construction and others. We have systematically assessed the degree to which material risk factors outlined by frameworks such as SASB and GRI are disclosed in key investor documents such as bond prospectuses. We have also assessed proxy statements such as SEC DEF14A disclosures to determine the degree to which companies have been linking executive compensation to decarbonisation targets. We have also used the tool to perform a baseline portfolio level assessment of disclosure levels and content for core TNFD indicators and metrics.
Certainly there are many other potential uses for automating analysis of unstructured disclosures and other content within sustainable finance. Please do contact us if you are interested in discussing a potential project or use case you have in mind.

AI-powered Large Language Models (LLMs) enable systematic Question and Answering capabilities comparable to many Active Ownership, Stewardship and Engagement processes.
Current and future advances that will further enhance our capability
Disclosure Assistant is already providing great results for customers searching and analysing disclosures and we are expecting the complexity and sophistication of those searches to increase as our Knowledge Base continues to mature and the volume and variety of disclosures grows.
Fortunately there are recent advances in the underlying technology which give us the opportunity to support the ever-increasing demand which is being placed on this type of engine.
LLMs that are specifically tuned and engineered to support retrieval-augmented environments are already starting to appear, based on academic work published only recently.
These LLMs provide both greater accuracy and more rapid results, even when answering questions where the answer has to be inferred from the known facts rather than being one of the pre-trained pieces of information.
Ready to drive sustainable growth in your business?
Contact us now to start your innovation & sustainability journey to arrange an exploratory conversation